Adapting the parametric uncertainty represented by the posterior distributions of the Bayesian estimate (see earlier notes) to the stochastic dynamic programming solution for the optimal policy. Simply requires evaluating the expectation over the distribution, but is computationally intensive given the spread and the three parameters.
f_transition_matrix <- function(f, p, x_grid, h_grid, sigma_g, pardist){
lapply(h_grid, par_F, f, p, x_grid, sigma_g, pardist)
}
par_F <- function(h, f, p, x_grid, sigma_g, pardist, n_mc = 100){
# Set up monte carlo sampling
d <- dim(pardist)
indices <- round(runif(n_mc,1, d[1]))
# Compute the matrix
F_true <-
sapply(x_grid, function(x_t){ # For each x_t
bypar <- sapply(indices, function(i){ # For each parameter value
p <- unname(pardist[i,c(4,1,5)]) # parameters for the mean, at current sample
mu <- f(x_t,h,p)
est_sigma_g <- pardist[i,2] # Variance parameter
if(snap_to_grid(mu,x_grid) < x_grid[2]){ # handle the degenerate case
out <- numeric(length(x_grid))
out[1] <- 1
out
} else {
out <- dlnorm(x_grid/mu, 0, est_sigma_g)
}
})
ave_over_pars <- apply(bypar, 1, sum) # collapse by weighted average over possible parameters
ave_over_pars / sum(ave_over_pars)
})
F_true <- t(F_true)
}
snap_to_grid <- function(x, grid) sapply(x, function(x) grid[which.min(abs(grid - x))])
# internal helper function
rownorm <- function(M)
t(apply(M, 1, function(x){
if(sum(x)>0){
x/sum(x)
} else {
out <- numeric(length(x))
out[1] <- 1
out
}
}))
Run the Bayesian analysis to obtain posterior distributions for parameters.
Test case using perturbed parameters
First, sanity test. Use the correct parameter values (slightly perturbed).
pardist <- mcmcall
pardist[,1] = p[2] + rnorm(100, 0, 0.000001)
pardist[,4] = p[1] + rnorm(100, 0, 0.000001)
pardist[,2] = sigma_g + rnorm(100, 0, 0.000001)
pardist[,5] = p[3] + rnorm(100, 0, 0.000001)
Compute optimal policy
sdp = f_transition_matrix(f, p, x_grid, h_grid, sigma_g, pardist)
s_opt <- value_iteration(sdp, x_grid, h_grid, OptTime=1000, xT, profit, delta)
Compare to the case without parameter uncertainty (growth noise only)
SDP_Mat <- determine_SDP_matrix(f, p, x_grid, h_grid, sigma_g)
pars_fixed <- value_iteration(SDP_Mat, x_grid, h_grid, OptTime=1000, xT, profit, delta)
Plot results
require(reshape2)
policies <- melt(data.frame(stock=x_grid, pars.uncert = x_grid[s_opt$D], pars.fixed = x_grid[pars_fixed$D]), id="stock")
ggplot(policies, aes(stock, stock - value, color=variable)) + geom_line(alpha=1) + xlab("stock size") + ylab("escapement")
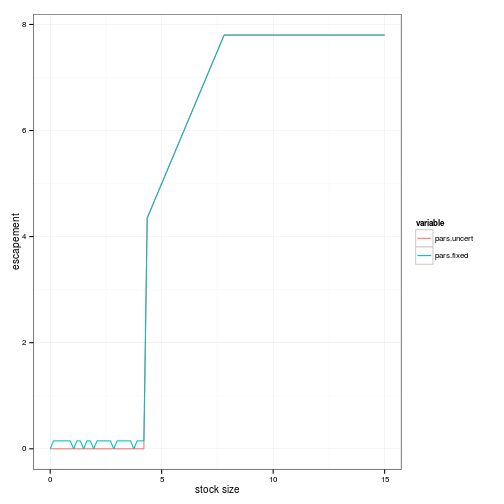
Using actual estimates
pardist <- mcmcall
Transform parameters back
pardist[,4] = exp(pardist[,4])
pardist[,5] = exp(pardist[,5])
Compute optimal policy
sdp = f_transition_matrix(f, p, x_grid, h_grid, sigma_g, pardist)
s_opt <- value_iteration(sdp, x_grid, h_grid, OptTime=1000, xT, profit, delta)
Compare to the case without parameter uncertainty (growth noise only)
SDP_Mat <- determine_SDP_matrix(f, p, x_grid, h_grid, sigma_g)
pars_fixed <- value_iteration(SDP_Mat, x_grid, h_grid, OptTime=1000, xT, profit, delta)
Plot results
require(reshape2)
policies <- melt(data.frame(stock=x_grid, pars.uncert = x_grid[s_opt$D], pars.fixed = x_grid[pars_fixed$D]), id="stock")
ggplot(policies, aes(stock, stock - value, color=variable)) + geom_line(alpha=1) + xlab("stock size") + ylab("escapement")
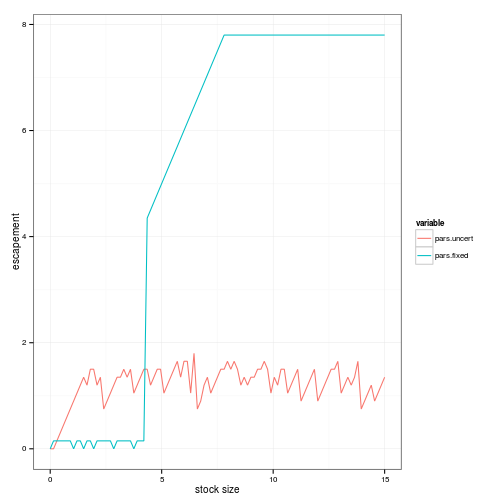
To Do
So far this is just a proof of principle example.
- Needs to be adjusted to account for uncertainty in the estimates of the noise processes as well.
- Needs to be written as generic and documented functions, add to nonparametric-bayes package routines.